
|
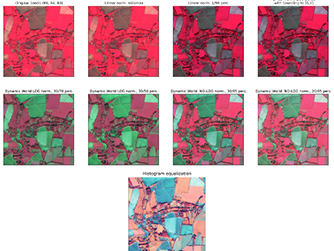
|

|
How to Co-Register Temporal Stacks of Satellite Images |
How To Normalize Satellite Images for Deep Learning |
Area Monitoring: How to train a binary classifier for built-up areas |

|

|

|
A Deep Learning Approach for Crop Type Mapping Based on Combined Time Series Of Satellite and Weather Data |
Challenges of large open-source datasets for building detection in Africa |
eo-grow - Earth Observation framework for scaled-up processing in Python |